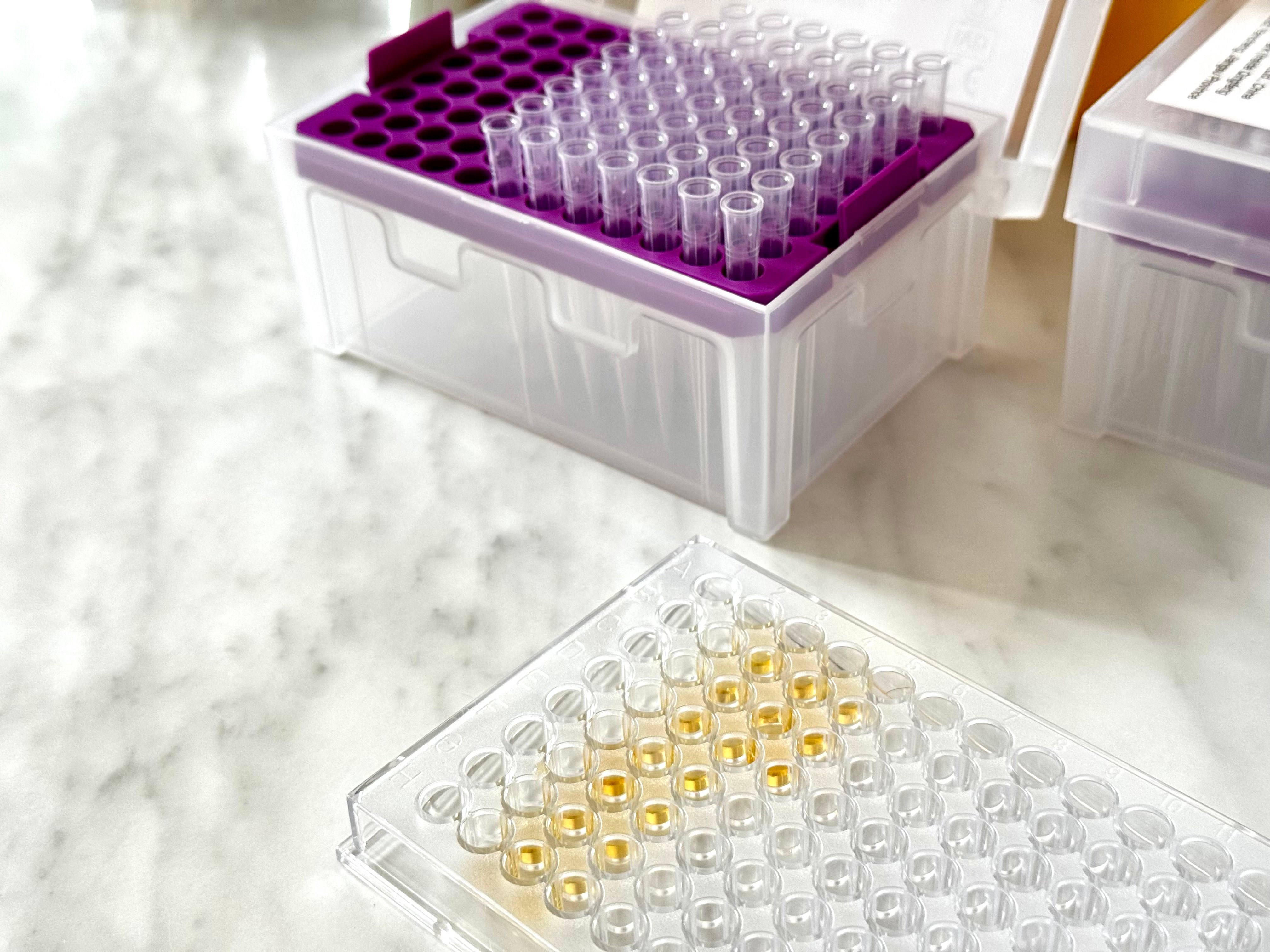
Developing High-Performance Antibodies Starts With Smarter Antigen Design

Antibodies are indispensable tools in life sciences, but their structure and subsequent performance depends on a critical first step: selecting the right antigen.
The process of antigen selection is often more of an art than a science, balancing structural feasibility and potential immunogenicity. Get it right, and you have a powerful reagent that binds its target with precision. Get it wrong, and you may end up with an antibody lacking fidelity to its intended target, latching onto anything but the protein of interest.
At Aviva Systems Biology, we have spent over 20 years generating antibodies using a variety of approaches to antigen design. By putting into place our new recombinant antibody pipeline, we have moved beyond traditional trial-and-error methods to a data-driven, AI-assisted strategy. Here is what we’ve learned about the challenges of antigen selection and how to best strategize and select the right antigen for antibody development.
The Antigen Design Puzzle
Once researchers identify a molecular target to develop an antibody against, the first challenge is to select an antigen that effectively prompts the immune system to produce high-affinity antibodies. Ideally, the antigen should mimic the target’s natural structure, ensuring that the resulting antibodies recognize the target in highly popular applications like immunohistochemistry, ELISA, or Western blotting.
However, predicting which antigens will succeed is not straightforward. Antigenicity assessments can help, but they often fall short of capturing the complexity of B-cell maturation and immune responses. Even when using predictive tools, researchers must balance a host of competing factors:
- Specificity vs. Immunogenicity – The antigen must trigger a robust immune response while remaining specific to the target.
- Expression and Solubility – The antigen needs to be easy to produce and dissolve in a format suitable for immunization, especially in large-scale manufacturing.
- Structural Integrity – If the antigen does not resemble the native protein structure, antibodies may recognize it in artificial settings but fail in real applications.
Why Full-Length Proteins Are Not Always the Answer
Using full-length proteins as antigens seems like a natural choice. They contain all possible epitopes and, if properly folded, can present appropriate conformational structures. But they come with major drawbacks. Large proteins are difficult to express, often contain hydrophobic or disordered regions, and may misfold in ways that do not resemble their native conformations. This can result in antibodies that recognize an artifact rather than the real target.
Peptides: Simple but Limited
Peptide antigens offer a more straightforward approach. They are relatively easy to synthesize, cheap to produce, and can be customized to target highly specific sequences. However, peptides have a critical weakness. They only present linear epitopes, ignoring the way proteins fold in three-dimensional space. This means that while peptide-generated antibodies may perform well in Western blotting, they often struggle in assays that require structurally-specific recognition, such as immunocytochemistry or ELISA.
The Protein Fragment Advantage
This is where protein fragments offer a compelling middle ground. Fragments are small enough to be easily expressed and purified but large enough to retain key structural elements of the native protein. If chosen correctly, they can provide:
- Easier expression and purification, reducing production bottlenecks.
- Better antigenic structure compared to peptides.
- Enhanced detection capabilities in real-world applications.
AI-Informed Antigen Design: Aviva’s Approach
Recognizing the limitations of traditional antigen design methods, Aviva Systems Biology has developed a proprietary AI-assisted strategy that refines antigen selection by integrating structural predictions with immunogenicity models. Our approach integrates:
- AI-Guided Antigen Propositions – Rapidly identify and rank potential antigen regions within target proteins.
- Structural Considerations – Predict antigen solubility and expression to improve production success rates.
- Antigenicity scoring – Assess the biophysical properties (amino acid charge, etc) of the selected propositions to determine the likelihood of robust immune response.
Example fragment antigen propositions from our NFκB test case. (Top) Three fragment regions were proposed (shown in green) from within the entire protein sequence (shown in blue). Below the propositions, our proprietary antigenicity scoring with proposition 2 sequence location highlighted. See Recombinant Antibody Discovery and Development: From Antigen Design to Characterization
This AI-assisted approach allows us to design antigen candidates that are not only immunogenic in vivo but also practical, ensuring better specificity, higher affinity, and more reproducible antibody performance in the end.
The Bottom Line
Antigen design is both an art and a science. Traditional approaches often force researchers to choose between full-length proteins, which are challenging to work with, peptides, which lack structural accuracy, and protein fragments, which require careful (and time-consuming) selection. At Aviva Systems Biology, we’re combining AI-powered insights with biophysical data to refine and improve the process, producing antibodies with higher success rates across applications (data to follow).
Aviva offers everything from off-the-shelf proteins to custom antigen design and antibody development, and the key is clear: better antigen design leads to better antibodies. No matter your need, We Have Your Covered.
Table 1. Advantages and disadvantages of different antigen types.